
What's Up With Women's Health?
Melinda Hu's Data Project 2 for Wharton's OIDD 245 Course
As someone who’s passionate about women’s health issues, I noticed that still, women aren’t comfortable enough to talk about these issues, and data are not being collected on how women truly feel about their healthcare. Frankly, we don’t really know what women are saying about their health.

Especially since Trump’s election, many women have become increasingly concerned over their healthcare (e.g. losing coverage for sexual and reproductive health services under ACA, the recent gag rule, etc.). In general, from my readings and observations, I noticed that women are not really talking about the health issues that are truly bothering them.
After some digging, I also realized there aren’t much data on what women are actually concerned about and how that’s changed between pre- and post-Trump election. Thus, I wanted to make this the focus of my project. Although it’s unlikely that women feel uncomfortable expressing concerns out loud and in person, talking online is more accessible and in-the-reach. This “digital exhaust” might reveal some interesting insights.

Data Sources
My first main data sources were Reddit Posts in the Women’s Health subreddit, from the time period of 6 months before Trump’s election on November 8, 2016 to 6 months post-election (i.e. May 8, 2016 to May 8, 2017). I retrieved these subreddit post links through a website called redditsearch.io since Reddit does not allow you to search by date, while redditsearch.io does.
I scraped these links using WebScraper. To do so, I first generated the correct Start URLs for the sitemaps (needed for WebScraper) by creating loops in R to (1) convert dates to epoch time in order to (2) create all the URLs for the results pages per month on redditsearch.io. I then scraped the content of these subreddit posts using RedditExtractoR.
My second data source was Google Trends, accessed via gtrendsR (will discuss more in detail in that section of the project).
To clean the Reddit data first, I limited the posts to individual user posts and created additional dataframes for before and after the election to do more specific analysis on each time period.

In total, after cleaning the data, I was able to scrape about ~800 URLs after cleaning. Since the URLs repeated for each comment in response to a post, this 800 number means 800 total pieces of text, which includes posts and comments.
To get a sense of the activity on this subreddit, I looked at the number of posts, number of comments, and upvote proportions.
(1) Even though I divided the time periods evenly at 6 months each, there were more posts after the election than before. Pre-election, there were 295 posts/comments, while post-election, there were 516 posts/comments.
There might’ve been multiple factors that affected this uptick in posts and comments, with the political environment being just one of them.
(2) Next, I looked at comments to see the kind of engagement these posts achieved. Pre-election, the mean number of comments was was 3.88, while the median was 3. Post-election, the mean number of comments was was 4.44, while the median was 3.5.
Though I can’t make any definite conclusions, I found it interesting that activity might’ve increased post-election. I speculated: did women feel more comfortable to be vocal online after the election? Maybe!
(3) Last to gauge how “supportive” this subreddit might be, I looked at the upvote proportion. Pre-election, the mean upvote proportion was 0.85, while the median was 1. With a median of 1, it might suggest that most people tend not to downvote posts. Post-election, the mean upvote proportion was 0.833, while the median was 0.81. This median suggests that on average, posts post-election were downvoted more (not a definite conclusion, but it’s interesting to note).
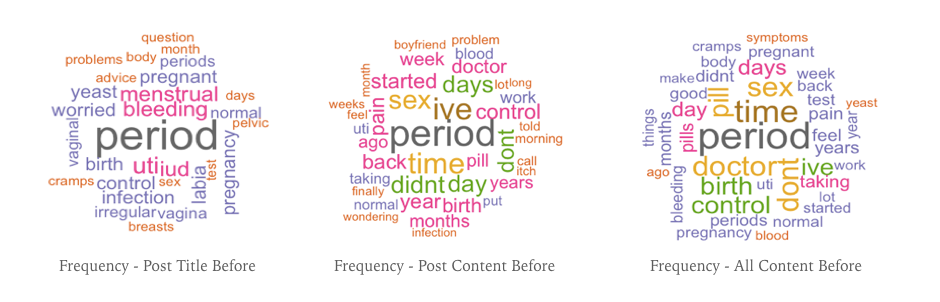
Frequent Words: Pre-Election
Now, to start exploring the text, I took the pre-election Reddit data and started some simple text analysis. I split my analysis into (1) the Reddit post titles (what you’d see scrolling through the main page “Women’s Health” subreddit. I saw the title like an email subject line - it’d pique readers’ interest and reveal the theme of the post; (2) the actual post content (further explanation by the author) and (3) all text, including the post title, content, and comments in response.
As RedditExtractoR repeats post titles and post content with each comment, I created separate dataframes for each of the 3 “text groups".” After creating a text corpus and document term matrix for each, I created wordclouds.
Immediately, you’ll notice that the topic of periods, a.k.a. menstruation, seems to appear most frequently across the board. (Side note: this pleased me, as I was working with the company #PeriodPainFree this semester. Women seem to be quite concerned about periods!)
Other patterns I noticed include how the post titles had words concerning to problems these women were worried about or had questions about, while the post content had more details into the problem (e.g. words related to time). Other common words allude to posts on bleeding, pain, birth control, sex, and other health problems they personally experienced (and seem to need help about).

I listed the frequencies with the top words for each text group, too. I ended up keeping words like “I’ve” and “don’t” even though I removed stopwords because it gave me some context as to how authors framed their posts (e.g. discussing personal experience, negative words).
In post titles, the top 5 words were directed at specific problems: periods, IUDs, UTIs.
In post content, these words suggest that short background stories are being told about this problem perhaps (e.g. words like days, started).
In all content, which adds in comments, we still see periods as most topical, with other words that weren’t frequent in the other two groups, like “doctor,” “pill,” and “birth control” (maybe these words are related to suggestions/advice by commenters).

Frequent Words: Post-Election
Repeating this process with the data from the post-election period, I first noticed that period was not the main word in all text groups.
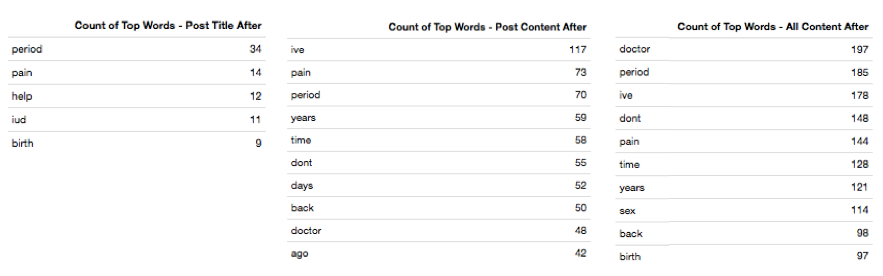
Nonetheless, periods still remained a top word: within top 3 most frequent for all 3 groups.
Post titles: the top 5 words were again related to specific problems, with more titles than before mentioning “help” and “pain.” Looking at the wordcloud, I saw numerous sexual and reproductive health terms pointing at certain problems.
Post content: The time-related words stand out once again, compared to the other two text groups. I noted that “pain,” which was not on any of the top words lists for pre-election, was the 2nd most frequent here.
All content: Compared to pre-election, the only different words are: “years,” “back,” and “pain.“ (Were there more people complaining about back pain for years, perhaps? 🤔️) I noted that “doctor” became more popular and that “pain” also appeared here (and didn’t before in pre-election).

Sentiment Analysis: Pre-Election
Just from seeing the above words, I imagine the stress that many of these women may have experienced when posting their stories or questions on the subreddit. Thus, using the “syuzhet” method/package, I did some sentiment analysis on the titles, post content, and all content. I also used the “formattable” package to include a gradient of the summary table, which would show the relative sentiment, a.k.a. from most to least negative in that particular text group.
Post title: The sentiment here is slightly negative, with a mean of -0.29 and median of -0.25. The summary also shows that a majority of post titles (75%, since the 3rd Quartile is 0) are negative, but not too negative (minimum is -2.5).
Post content: The sentiment here is slightly more negative than the titles, with a mean of -1.025 and a median of -0.575. The summary also shows us that there's a wider range of positive and negative emotion, with a lean towards more negative (minimum at -21.8 versus maximum at 6.1).
All content: The sentiment in all content - post title, post text, and comments - is slightly more positive than just post title and post text alone, with mean of -0.0214 and median of 0. One possibility is that people are responding in a more positive and encouraging manner in the comments.

Sentiment Analysis: Post-Election
Before looking at the post-election sentiment, I hypothesized that it would be more negative. Some say that Trump has made Americans more negative and stressed. Could that have been reflected a bit in these posts?
Post title: Once again, the sentiment in titles is slightly negative, with a median of -0.2500 and a mean of -0.3161, about the same as titles pre-election. The spread of emotions is small, with a minimum of -2.65 and a maximum of 1.35.
Post content: The sentiment here is slightly more negative than the titles (similar to pre-election), with a median of -0.4000 and mean of -0.8521. This is pleasantly surprising - these posts are a bit brighter than those pre-election. The summary also shows us that there is a more balanced range of positive and negative emotion, with a minimum at -7.25 and maximum at 7.1.
All content: All content is slightly more positive than just post title and post text alone, with a mean of -0.01067 and median of 0. This is similar to what I saw pre-election. I'm glad the comments reduce the negative sentiment a bit. ❤️
Overall, it seems to me that the post titles, post content, and overall content with comments tend to be a little negative, neutral at best. There doesn’t seem to be a large difference between pre- and post-election sentiment (unlike what I hypothesized).

Topic Models
Using the the “topicmodels” package with “Gibbs method,” I also tried to determine the main topics discussed in these posts. Admittedly, the words here aren’t all pointed at a specific topic… Thus, I limited it to 3 topics with 8 words each.
Before Election: The 3 main topics seem to be about (1) periods/menstruation (2) doctors/birth control (3) sex.
After Election: The 3 main topics seem to be (1) sex (2) periods/birth control (often used to regulate periods), and (3) doctor/symptoms (everything else it seems).
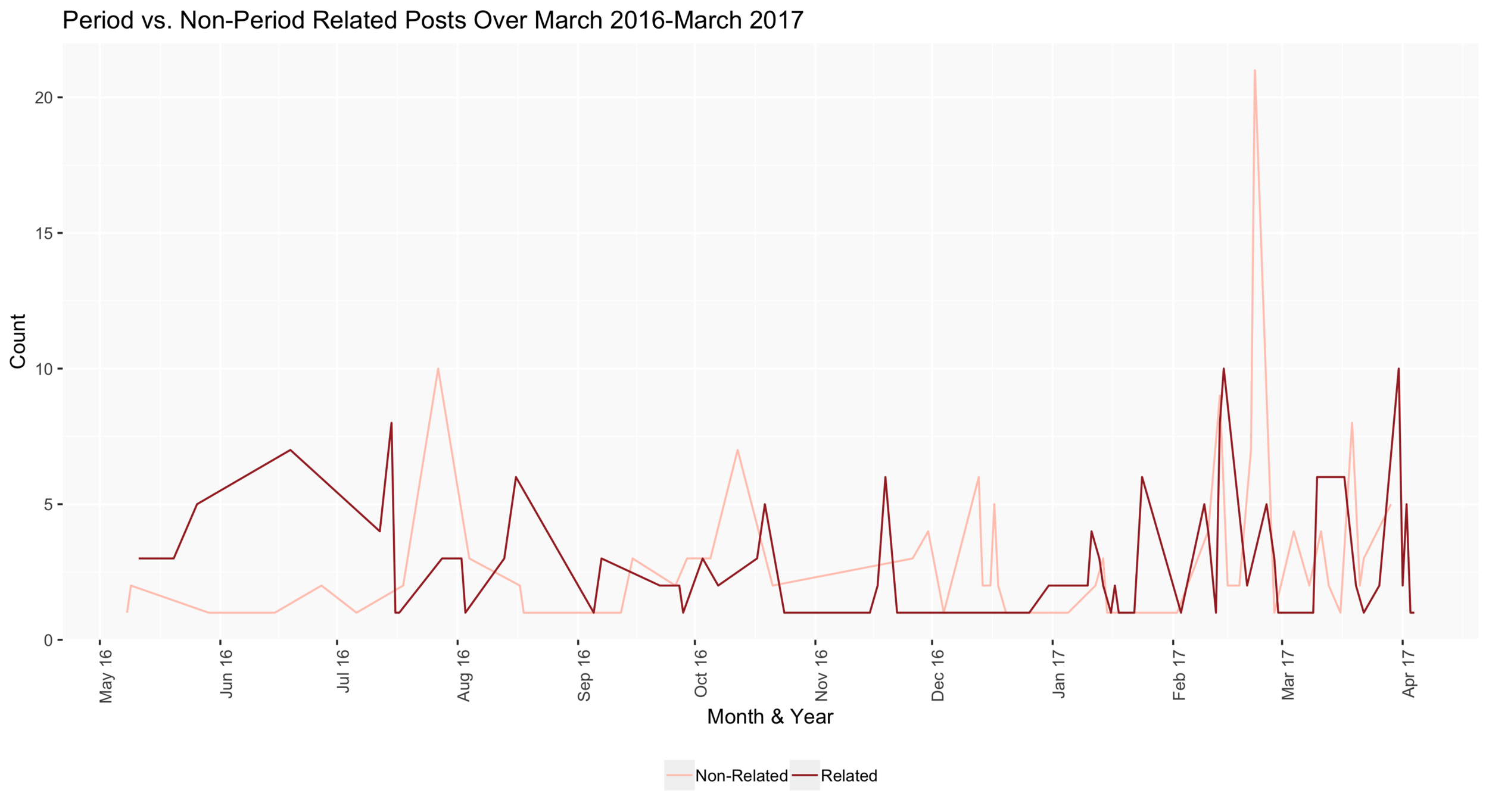
Trend Over Time: "Period"
I looked back at the top words, wanting to dive a bit deeper into how mentions of these words changed over the full year, pre- and post-election.
The top 10 words pre-election were: period, time, doctor, dont, sex, pill, birth, control, ive, days. The top 10 words post-election were: doctor, period, ive, dont, pain, time, years, sex, back, and birth.
The 4 words I bolded-period, doctor, sex, and birth-were in the top for both time periods, so I decided to look into those.
First, from the initial full year dataset to create the graphs, I created smaller dataframes, using conditional statements and summarize/group by statements to count the number posts that contained the word “period” in the post text.
Then, using the package '“ggplot2,” I plotted this count over time. There seemed to be blips every month or so, with slightly more activity in the first months of 2017, but no abnormal patterns. The blips seem to be in the middle of the month, but there are too few data points to say anything about that (plus, there isn’t evidence that women tend to get periods in the middle of the month, for example).
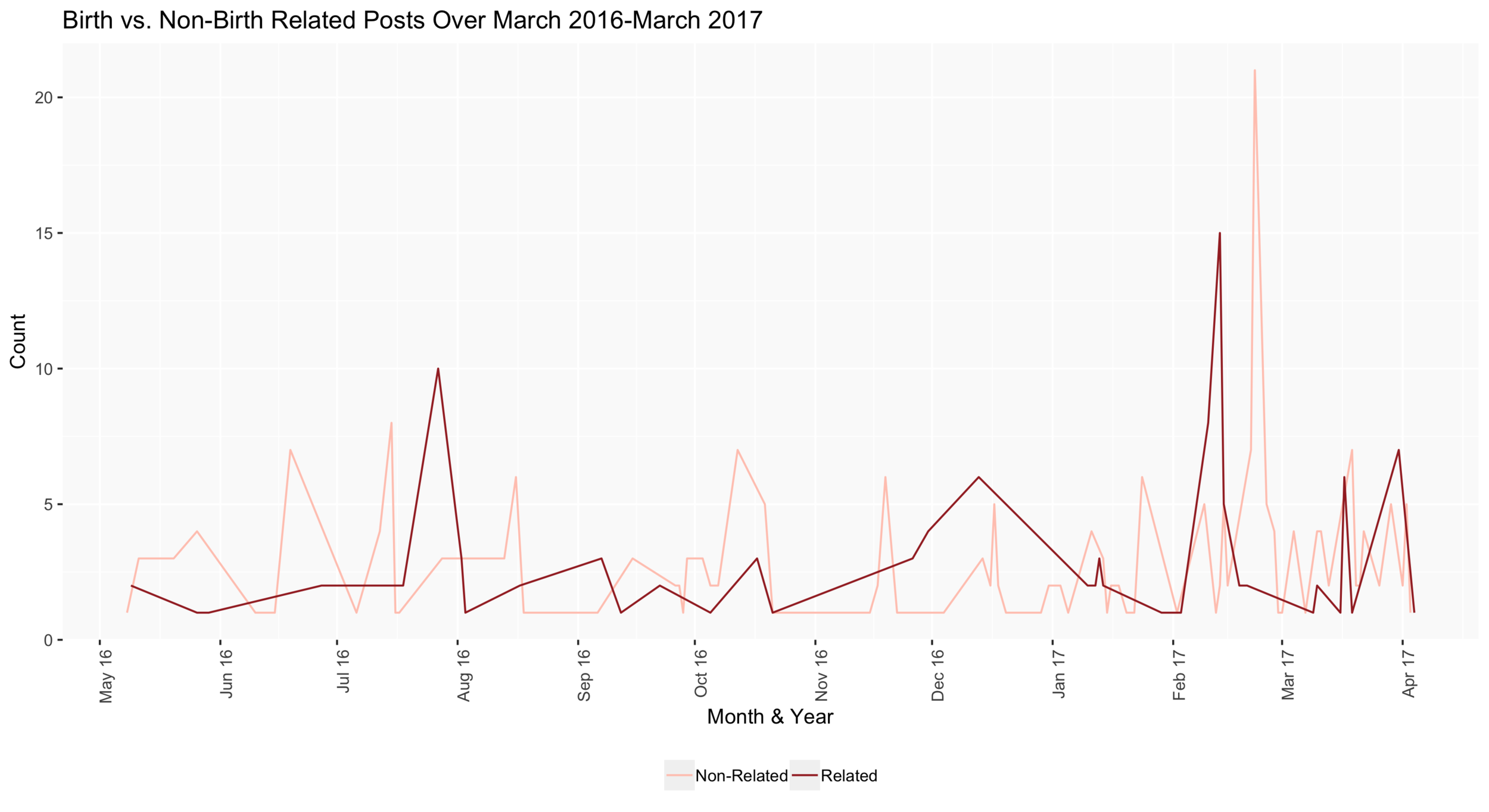
Trend Over Time: "Birth"
Here, there are two noticeable jumps in the number of posts mentioning “birth.” The bigger jump is in early February, which just so happens to be Valentine’s Day and around when the House passed a law that allowed states to withhold federal funding for health care providers that performed abortions (HJ Res 43). However, it’s hard to prove a connection to either factor from this small dataset.
Overall, the two lines - posts related to “birth” and those not related to “birth” - are generally moving up and down alongside each other. In other words, even if a month has more mentions of “birth,” it’s likely because in total there were more posts that month (i.e. more posts on non-related topics too).

Trend Over Time: "Sex"
Again, here, there also doesn’t seem to be a noticeable pattern. I did, however, note that there’s a large increase in posts in late February 2017 in non-related topics…
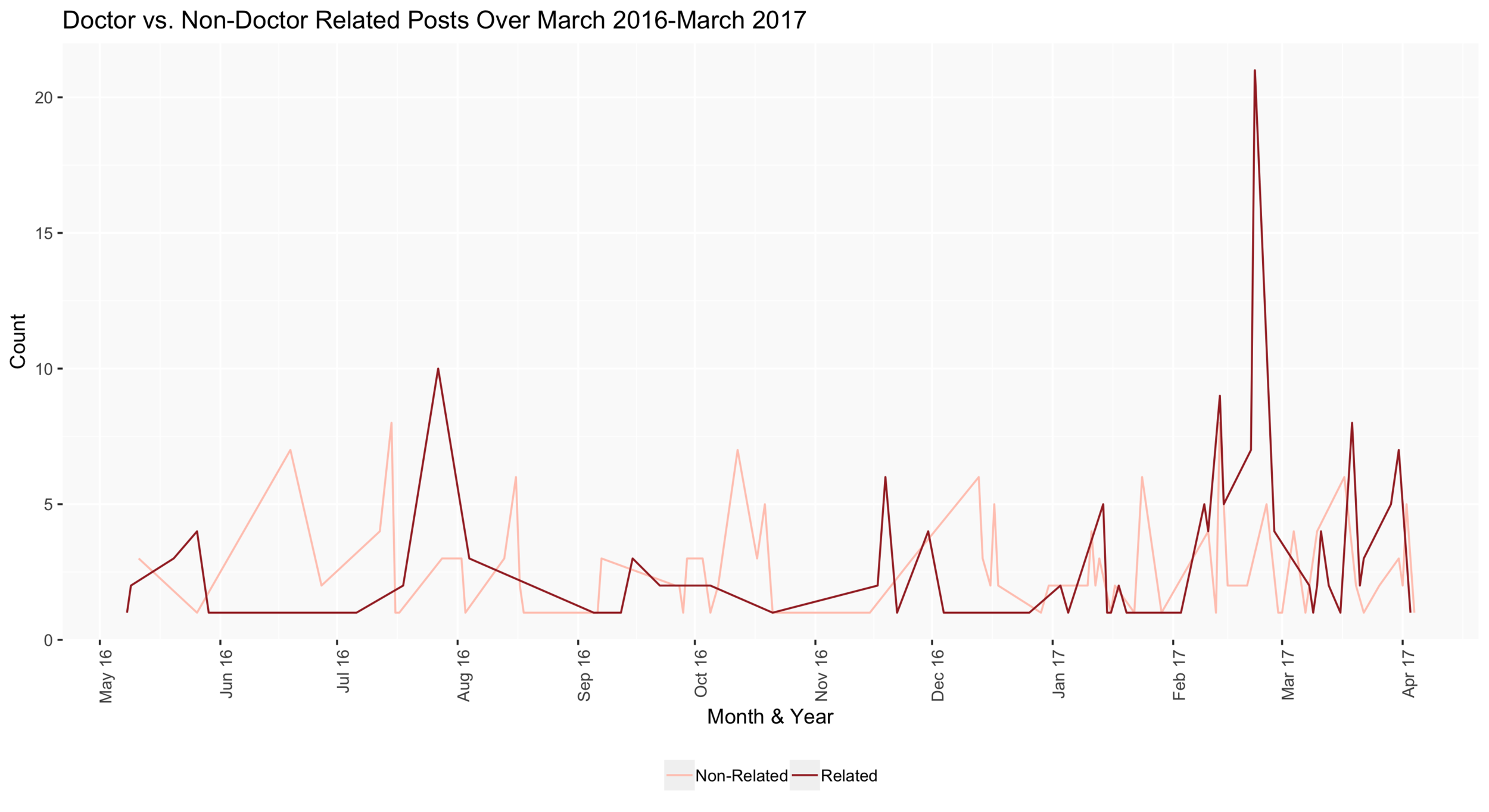
Trend Over Time: "Doctor"
There was a noticeable blip in late February 2017 with posts related to doctors. Otherwise, there isn’t a noticeable pattern.
Overall, though I was excited at first, there isn’t an obvious trend on the frequency these four words are mentioned over time. From the graphs, it seems like there was slightly more activity on the subreddit in 2017, but since the election was in November, it’s unlikely that the election affected the mentions of certain words.

Correlation Between Words and Comments
Finally, I wanted to see which words seemed to generate comments, or in other words, which words mentioned in the post title were correlated with more comments.
Before Election: The top 10 words associated with “respondable” posts, which I deemed as those with more than 4 comments, are: "antidepressants", "denied", "legal", "new", "rape" "recurring" "support", "oil", "tea", and "tree" (not sure why “tree” is there…) It was interesting to note the provocative words (e.g. “denied,” “rape,” “antidepressant”), which didn’t appear in the top frequency lists but generated a more than average number of comments.
After Election: The top 10 words associated with “respondable” posts were: "fatigued", "days", "why", "hair", "bra", "eczema", "gut", "leaky", "nipple", and "stain." I’m surprised that no words overlapped. Could it be that after the election, people felt less comfortable responding to more serious topics and preferred more everyday topics related to hair, bras, and fatigue?
Again, I recognized the possibility that my sample size was too small to draw any strong conclusions about the change in correlations pre- and post-election.
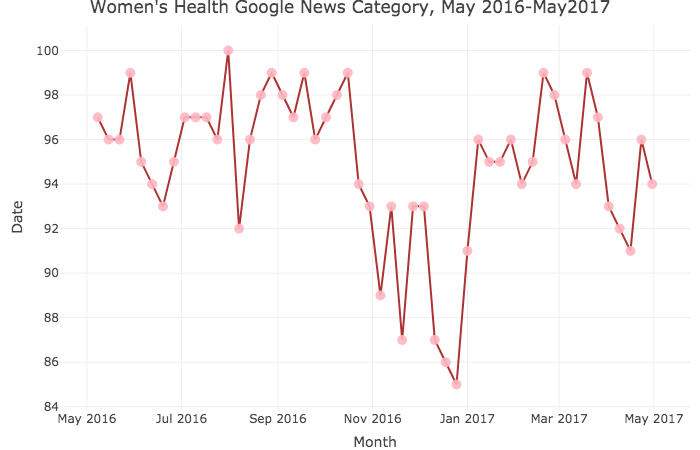
Google News Category - Women's Health
Aside from Reddit, I saw Google as another place to gather information on “digital exhaust” relating to women’s health. I wanted to see if the popularity of women’s health news topics matched the activity in the Women’s Health subreddit.
So, using the “gtrendsR” and “plotly” package, I created a few graphs. Narrowing it down to Google Trends category 648, a.k.a. “Women’s Health,” and just the “News” category, I saw that there was actually a dip in articles on women’s health after the election. This trend wasn’t reflected in the previous graphs on posts in the subreddit (no noticeable drop in either related or non-related posts during that time frame).
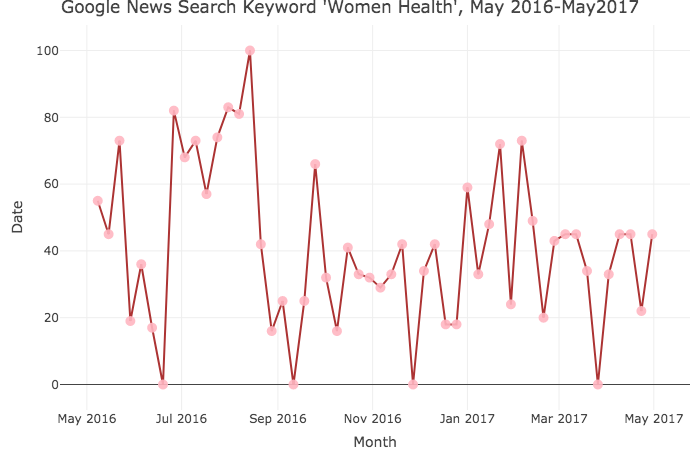
Google News Search - Women's Health
As not all women’s health articles might’ve been categorized as “women’s health” by Google, I also looked at the number of hits for a “Women’s Health” search in Google News. There seemed to be a slight pickup in articles mentioning women’s health in the summer before the election. Otherwise, there wasn’t any abnormal pattern, which matches what I found in the subreddit posts.

Google Web Overall - Women's Health Category
Increasing the scope of results to Google Web overall, I again observed this drop in women’s health-related content in the month or two after the election. It’s possible that fewer pieces of content created / fewer searches categorized as women’s health during the holiday season (that Trump was elected was not a motivation for more searches). It’s interesting to see that this drop didn’t reflect in the subreddit.

Google Web Overall - "Women's Health" Search
Similar to the previous chart, there’s a drop in searches for “Women’s Health” in December 2016 to January 2017. But, I do notice a higher number of hits for a “Women’s Health” search in 2017 than in 2016. This pattern was very slight in the subreddit post graphs.
All in all, I hoped that the gtrendsR package and Google Trends data could provide some context for women’s health-related content. That Google Trends matches the Women’s Health subreddit in that there is not a clear trend between pre- and post-election is informative!
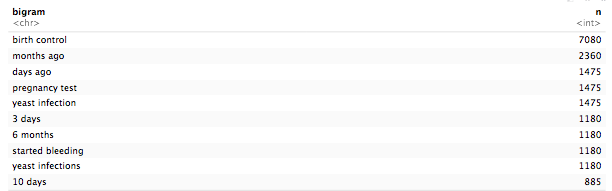
Future...
In the future, I hope to do better text analysis with phrases and groups of words. I discovered packages related to “bigrams” really late into this data exploration process, so I didn’t get a chance to re-do my analysis. (Image above is a preliminary screenshot of popular two-word phrases.)
I also hope to scrape and/or discover a more robust data set on the online posts and conversations that women are having related to their health. Initially, I had tried to scrape women’s health forums, but the scraping process was extremely difficult. Ideally, i’d be able to incorporate the conversations happening in all the sites in which women ask and respond to women’s health topics.
Last, I’d also hope to conduct more numbers-based analysis (e.g. regressions) so I can come to more confident and significant conclusions about the patterns I’m noticing in the data.
In the end, it seems that in the Women’s Health subreddit, there were not significant differences in the topics women were talking about, how they talked about them, and how frequently they talked them pre- and post-election of Trump. Google Trends seemed to support this insight as well. Nonetheless, I’m glad that I dug into a topic that (1) is extremely important to me and (2) hasn’t gotten much attention in terms of gathering data and uncovering insights. I’ll bring these learnings on what women are talking about online in regards to their health with me in my future endeavors.
Thank you!